Semantic Data Mapping
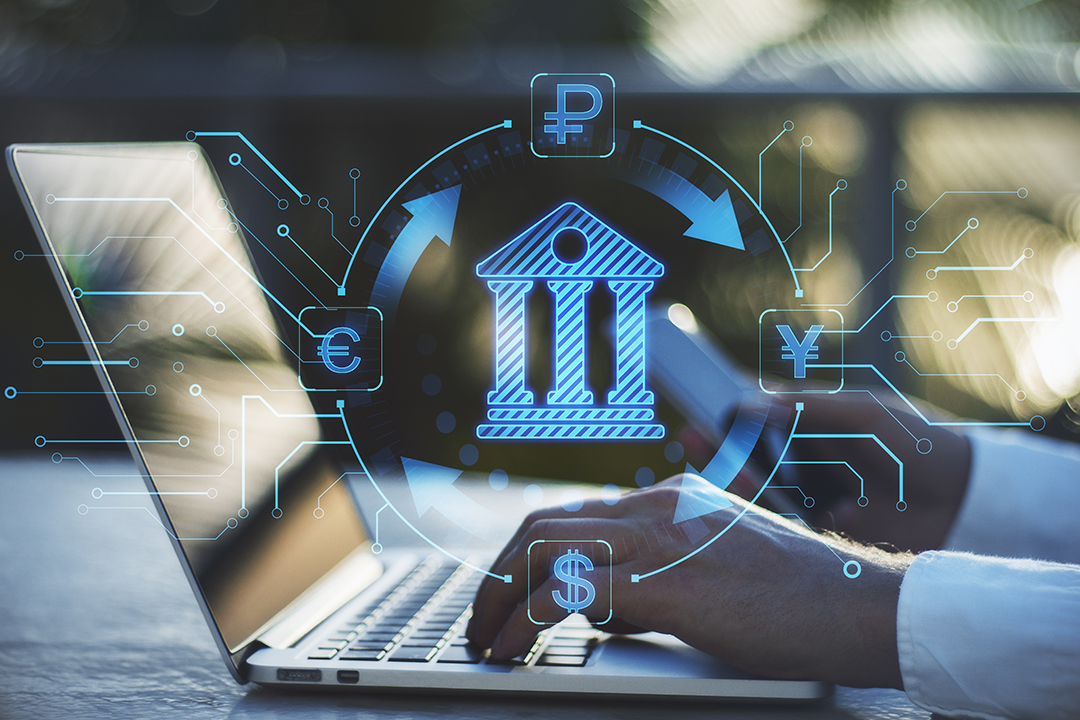
Semantic data mapping is the process of establishing relationships and connections between different data sources or systems using semantic web technologies. It involves mapping the data elements from one system to another in a way that allows them to be integrated and used together.
Semantic data mapping with GLIDE typically involves the following steps:
Defining the scope and objectives of the data mapping project: Identifying the data sources and systems that need to be mapped and defining the goals and objectives of the project.
Identifying the data elements and attributes to be mapped: This involves finding the data elements and attributes that need to be mapped from one system to another.
Defining the data mapping rules: Establishing the rules that will be used to map the data elements from one system to another.
Implementing the data mapping process: GLIDE provides a user interface that allows users to define the data mapping rules and to monitor the progress of the data mapping process.
Validating and testing the data mapping process: It is important to test and validate the data mapping process to ensure that the data elements are correctly mapped and integrated. GLIDE will manage and monitor this process.
Overall, semantic data mapping is an important process for integrating data from multiple systems and sources. It helps to ensure that data is consistent, accurate, and can be used effectively to drive insights and decision-making.
GLIDE aids in the transformation of data elements from one namespace into another namespace. Essentially the problems arising in semantic mapping are the same as in data mapping for data integration purposes, with the difference that here the semantic relationships are made explicit through the use of ontologies which play the role of data dictionaries in data mapping.
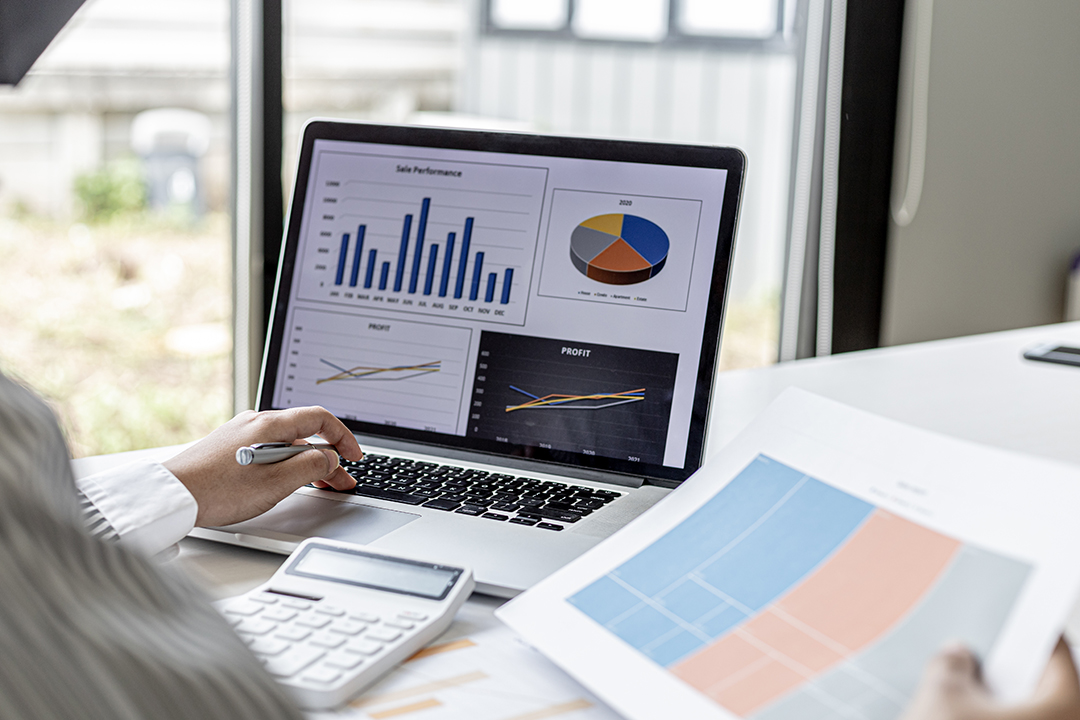
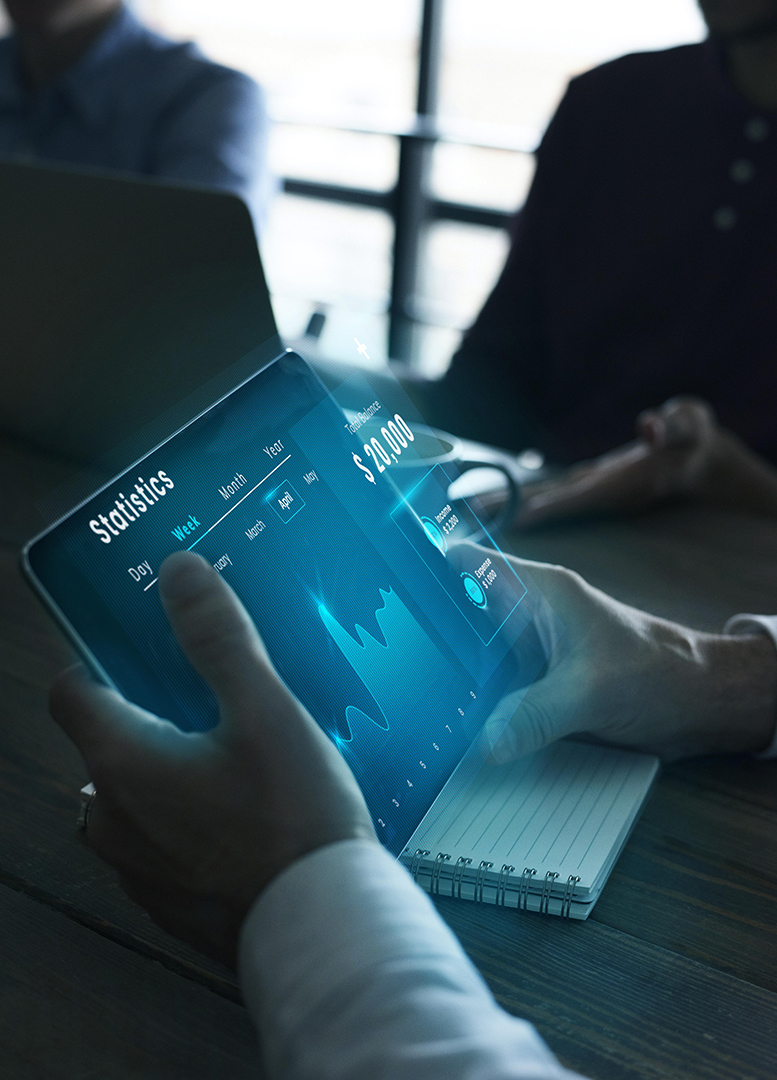

KNOWLEDGE GRAPHS AND DATA MAPPING
WHY USE A KNOWLEDGE GRAPH FOR SEMANTIC DATA MAPPING?
Knowledge Graphs are principally used for Data Inference and contextual data relationships. Data are stored as triples within Knowledge Graphs. Semantic Data Mapping is used for Data Lineage where attributes are intelligently connected across different systems using machine intelligence. With a Knowledge Graph driven Semantic Data mapping, it becomes all the more easy for the ML mapping to make accurate predictions on attribute and metadata lineage.
WHAT ARE THE KEY BENEFITS OF USING A KNOWLEDGE GRAPH FOR SEMANTIC DATA MAPPING?
A semantic mapper must have access to three data sets:
List of data elements in source namespace
List of data elements in destination namespace
List of semantic equivalent statements between source and destination
These concepts are also known as triples and these are persisted naturally in a Knowledge Graph.
A Knowledge Graph constructs a comprehensive view of nodes, edges, and labels through a process called semantic enrichment. When data is ingested, this process allows Knowledge Graphs to identify individual objects and understand the relationships between different objects. Once a Knowledge Graph is complete, it allows question answering and search systems to retrieve and reuse comprehensive answers to given queries, which in turn is used by the semantic data mapper.
WHAT SORTS OF COMPANIES NEED DATA LINEAGE AND SEMANTIC DATA MAPPING?
-
Data Lineage and Semantic Data Mapping are particularly needed by companies that have voluminous data, where accurate and deep insights and inferences are needed from their data. Lineage helps establish the linkages amongst various data attributes and tie these attributes across the organization & thereby help draw inferences.
-
Semantic Data Mapping is one way of establishing data lineage across the enterprise. In semantic data mapping, connections are made to various disparate data sources in the enterprise and through a ML based train & deduce algorithm, the data attributes are matched across various systems, and presented to the user for validation & confirmation by the machine.
USE CASES
Knowledge Graphs have applications in many industries, such as:
-
Retail: Knowledge Graphs are used for up-sell and cross-sell strategies, recommending products based on individual purchase behavior and popular purchase trends across demographic groups.
-
Entertainment: Knowledge Graphs are also leveraged for artificial intelligence (AI) based recommendation engines for content platforms, like Netflix, SEO, or social media. Based on click and other online engagement behaviors, these providers recommend new content for users to read or watch.
-
Finance: This technology is used for know-your-customer (KYC) and anti-money laundering initiatives within the finance industry. This assists in financial crime prevention and investigation, allowing banking institutions to understand the flow of money across their clientele and identify noncompliant customers.
-
Healthcare: Knowledge Graphs benefit the healthcare industry by organizing and categorizing relationships within medical research. This information assists providers by validating diagnoses and identifying treatment plans based on individual needs.
USE CASES
Knowledge Graphs have applications in many industries, such as:
-
Retail: Knowledge Graphs are used for up-sell and cross-sell strategies, recommending products based on individual purchase behavior and popular purchase trends across demographic groups.
-
Entertainment: Knowledge Graphs are also leveraged for artificial intelligence (AI) based recommendation engines for content platforms, like Netflix, SEO, or social media. Based on click and other online engagement behaviors, these providers recommend new content for users to read or watch.
-
Finance: This technology is used for know-your-customer (KYC) and anti-money laundering initiatives within the finance industry. This assists in financial crime prevention and investigation, allowing banking institutions to understand the flow of money across their clientele and identify noncompliant customers.
-
Healthcare: Knowledge Graphs benefit the healthcare industry by organizing and categorizing relationships within medical research. This information assists providers by validating diagnoses and identifying treatment plans based on individual needs.
ADDITIONAL RESOURCES
Resources from our recent webinar on “Semantic Data Mapping in the Context of Data Lineage”
NEXT STEPS
To learn more about how Trigyan can help you with your KYC project, click the button to contact us.